UP study identifies best models for predicting algal blooms
A new study by the University of the Philippines Diliman revealed two optimal models for predicting algal blooms, particularly in Laguna Lake, one of the sources of freshwater fish for Metro Manila and nearby areas.
An algal bloom is the overgrowth of algae in a body of water. The standard method for monitoring its population in water is measuring chlorophyll-a, the green pigment found in algae.
“If we wait for [the instruments] to indicate high algal content, it may already be too late since the bloom may have already occurred,” said author Dr. Karl Ezra Pilario of the UPD Department of Chemical Engineering.
Pilario's team found that monitoring nitrate and phosphate concentrations in the water is a more effective approach to predicting algal blooms.
Changes in these concentrations are often linked to increases in chlorophyll-a, and advanced tools such as machine learning models can be used to establish these complex relationships from data.
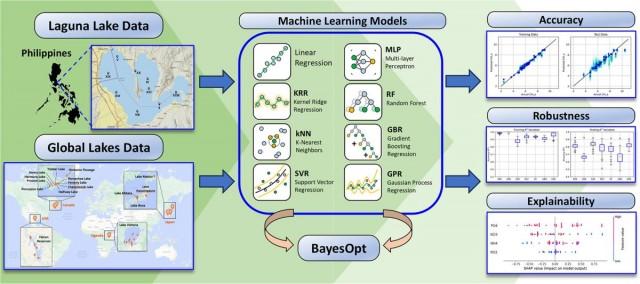
The study, which was published on ScienceDirect in December 2024, compared the robustness and accuracy of eight machine learning models using water quality data from Laguna Lake and historical data from global lakes.
Of these learning models, two were found to perform better than the other models: the Kernel Ridge Regression (KRR) and Gaussian Process Regression (GPR).
KRR was the most accurate for Laguna Lake, while GPR was the best for global lakes.
“Now that we have an accurate, robust, and explainable predictor of chlorophyll-a, we can deploy the model for rapid detection of impending algal blooms,” Pilario said.
“We recommend monthly monitoring of these values so that if an impending algal bloom is detected, there is ample time to prepare for interventions or mitigation strategies,” he added.
Researchers are also looking into incorporating additional predictors like weather conditions, land cover types, and other effects caused by humans.
They also plan to test the models with Laguna Lake samples taken at different times of the year.
"Lastly, on the modeling side, we need to test more models that might be more accurate than KRR or GPR. In the future, we encourage researchers to test for the robustness and explainability of their machine learning models, and not just for accuracy, because it helps make the results more believable for policy-making,” Pilario said. — VBL, GMA Integrated News