How UP mathematicians detect potential stock market crashes
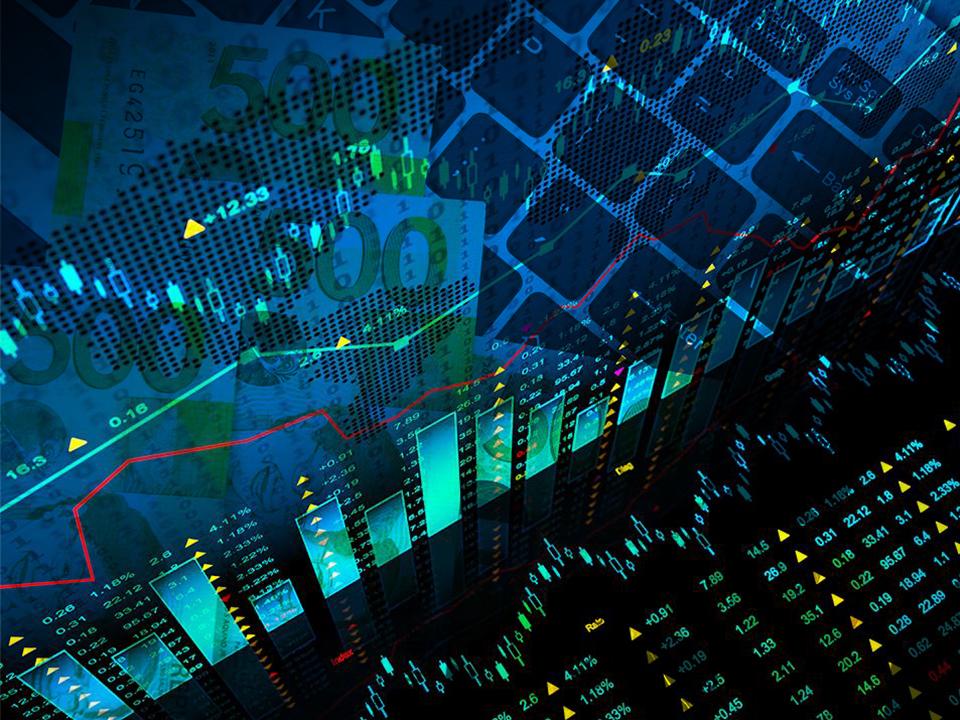
Mathematicians from the University of the Philippines Diliman have found a new approach to analyzing stock market movements.
Topological data analysis (TDA), which finds patterns in large datasets, was utilized by Ela Mae Riñon and Dr. Rachelle Sambayan of the UP Diliman College of Science Institute of Mathematics to predict when the stock market was about to crash.
Specifically, the researchers applied TDA to the January 2019–January 2021 stock price data of three Philippine companies: Century Pacific Food (CNPF), PAL Holdings (PAL), and Cebu Air (CEB).
''To understand TDA, it may be helpful to compare it with how we observe stars. At first glance, the night sky appears as a seemingly random plot of stars scattered across the vast canvas of the universe. However, with extended observation, patterns emerge: clusters of stars, constellations, and voids become apparent the moment we recognize them. As we continue to gaze at the sky, the random stars fade into the background, while the clusters, constellations, and voids take the spotlight,'' the UPD-CS said in a press release on Tuesday.
''This effect is similar to what TDA does with large sets of data points. Essentially, TDA reveals hidden geometric structures within datasets, allowing insights to be drawn from what previously looked like an ambiguous clump of points.''
In this case, the researchers classify the figures into three homology groups: connected components, which are open shapes like line segments; loops, which are closed shapes like triangles; and cavities, which are shapes that extend to the third dimension.
''They found that when the market was about to crash, the data points began to cluster together, which made their corresponding figures more likely to change homology groups. In other words, the persistence of homology groups weakens as the stock prices start to plummet,'' the UPD-CS said.
“This weakening of persistence is unexpected because it reveals a distinct change in the data's topological structure during market downturns, which contrasts with the scattered point clouds observed during stable periods,” the authors added.
Unlike CEB, both PAL and CNPF were more stable during the onset of the COVID-19 pandemic (early 2020), which led to travel bans and lockdowns, the study found. Although PAL and CNPF experienced minor dips during this period, they did not experience a significant crash, which the model correctly anticipated.
The authors suggest including other markets and time periods in future studies.
“Another avenue for future research is applying the TDA approach to other types of time series data, such as exchange rates, to explore its effectiveness in detecting structural changes and understanding the behavior of stock prices and other financial indicators under different economic conditions,” they said.
The study was published in the Philippine Journal of Science in June 2024. —VBL, GMA Integrated News